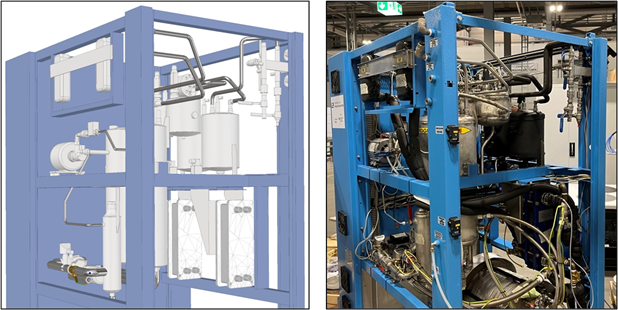
Engineering assistance for hybrid decision support based on machine learning surrogate models
This project aims to transfer MLS approaches from analysis tasks related to physical phenomena, e.g., static loads or fluid dynamics, to the analysis of later product creation phases like manufacturing and assembly. Design phase decisions are driven not only by a product or component’s performance but also by the impact on downstream processes. In particular, effort, associated costs, quality, and sustainability metrics such as energy demand or carbon footprint. If the analysis of an envisioned solution is carried out instantaneously within the design process and provides insights from subsequent processes, product developers receive direct feedback on the consequences of their decisions. This enables proactive effort reduction in later stages of product creation by allowing designers to influence production criteria. For example, time, costs, and resources can be saved by rationalizing production steps, adapting assembly orders, or designing for automation. In addition, products that are easy to manufacture are generally also easy to dismantle or repair, which leads to additional savings during the product use, maintenance, and recycling phases. Thereby, the intended results of this project provide a hybrid decision support for engineering design and form the methodological basis for the implementation of corresponding assistance functionalities in CAD and other digital engineering tools.
The approach to be pursued in this project is to use lightweight approximating simulations using MLS to examine and assess the current status of a specific design concerning the implications in terms of the spatial layout of the main components, the assembly sequence and the ability to automate the assembly process, thereby highlighting weaknesses and providing up-front validated feedback and potential alternative design options for design engineers. In the context of the planned research work, the example of the engineering design and assembly process of electrolyzers is used for the problem definition and the validation of the research results.
The project will be divided into two research lines, each focusing on three overarching research questions.
RQ 1: What are the key requirements for engineering assistance functionalities that can effectively support assembly-relevant decisions in engineering, taking into account spatial and layout considerations, assembly sequencing, and automation possibilities, and how can these requirements be generalized across various product development contexts?
RQ 2: How can RL be utilized within a virtual simulated environment to develop a computational model that enhances spatial configuration and layout-oriented assembly design decisions, and what are the optimal strategies for defining the states, actions, and reward/penalty structures to train the RL agent effectively for diverse and evolving assembly requirements?
RQ3: How can MLS-enabled engineering assistance be generalized for application in digital engineering tools, and what are the implications for engineering methods and procedures, particularly in terms of user acceptance, human-machine interface design, and the provision of instant or real-time feedback within a CAx environment?
RQ4: How can the most important factors for automation capability concerning product design be defined, and how these can be weighed against each other?
RQ5: Which preconditions/constraints must be set/defined to ensure a feasible result and limit the computational effort?
RQ6: How can local optima be avoided when evaluating an automated assembly with a created evaluation metric?
The structure of the work program, including both research lines, is depicted in the following figure.
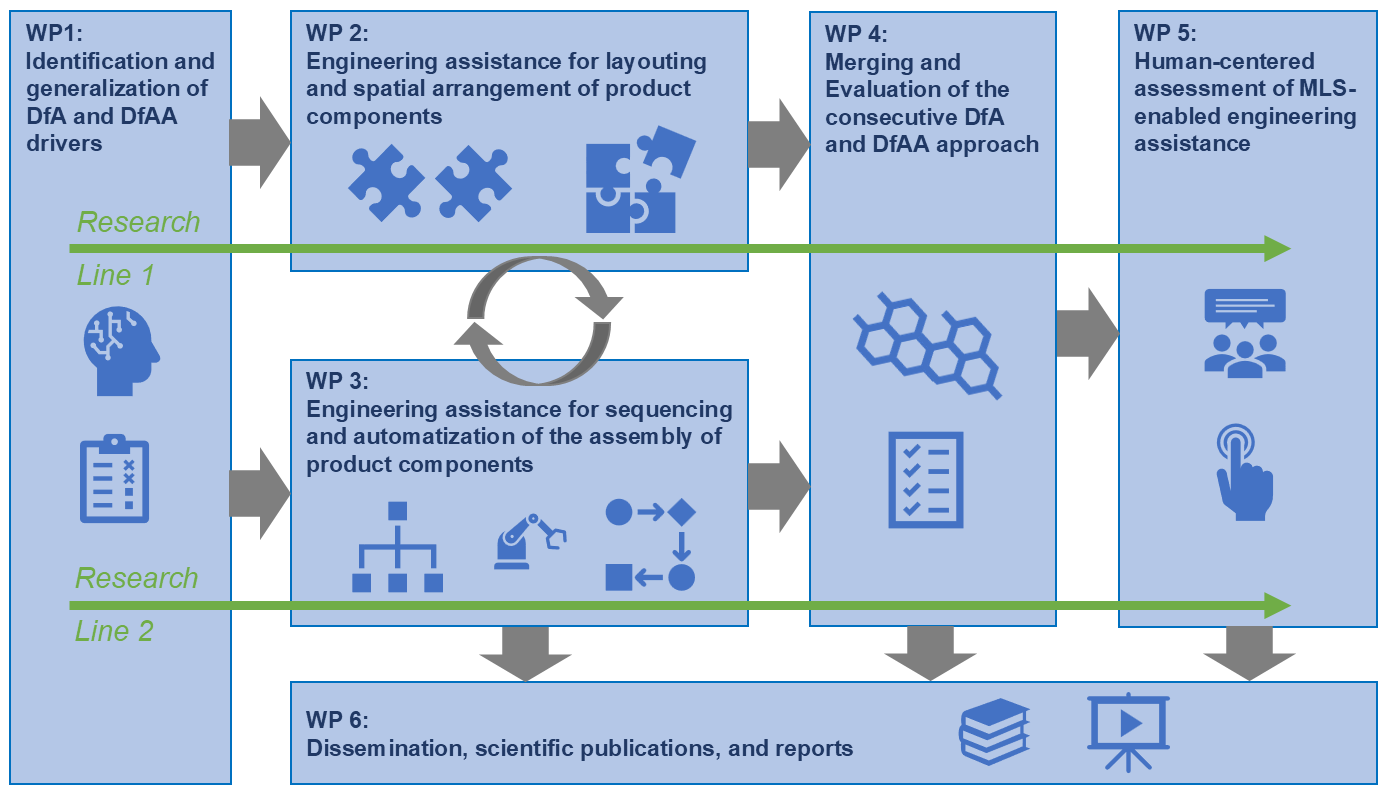